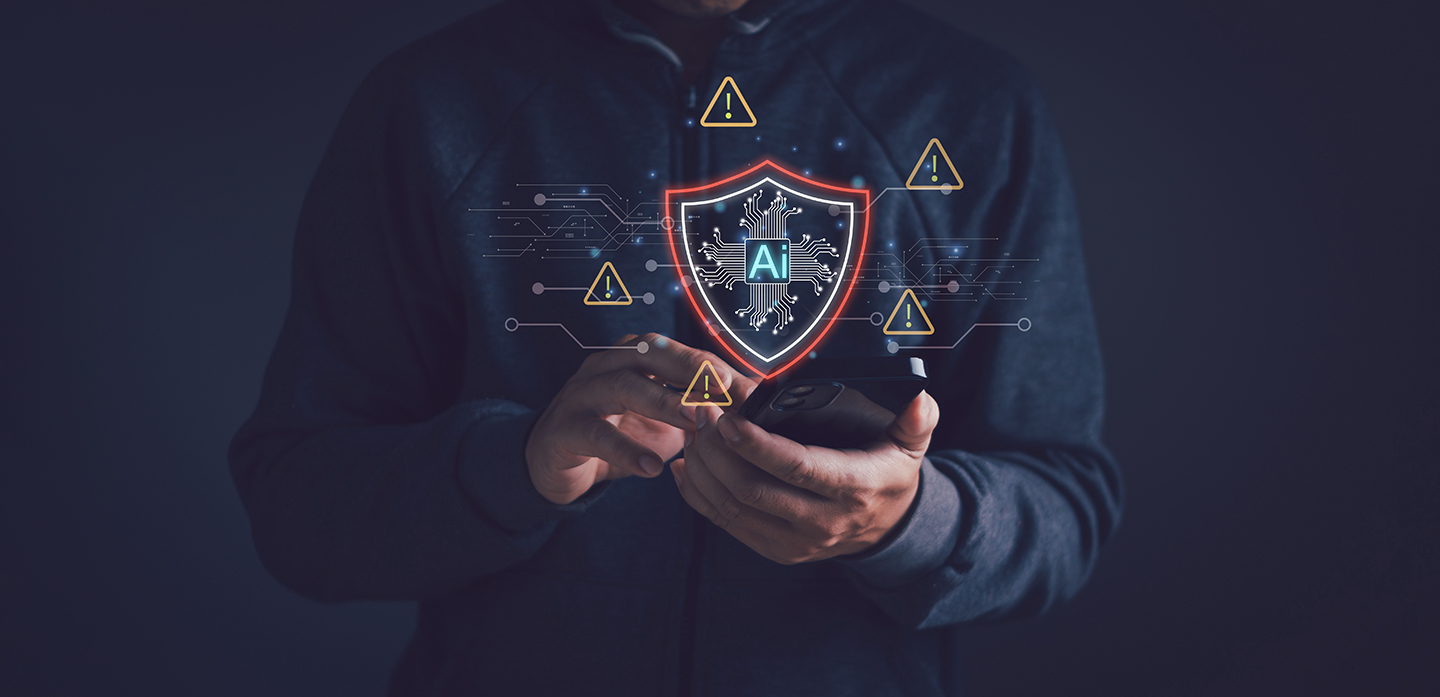
AI and Data Protection: How to Protect Your IP While Using AI
AI and Data Protection are top priorities for any organization diving into artificial intelligence. Whether you’re already leveraging AI or just starting to explore it, you’re likely feeling both excitement and concern. And that’s very common.
AI’s capabilities are phenomenal; it can streamline operations, unearth insights you didn’t know existed, and even predict future trends with impressive accuracy. But as you deepen your involvement in the AI world, one major question looms:
“How can I protect my intellectual property (IP) in this AI - driven ecosystem?”
I've been there myself. Balancing the thrill of AI’s possibilities with the need to protect IP is a unique challenge, especially when sensitive data and proprietary information are on the line. Let’s explore why IP protection is so crucial in the AI era and look at some strategies to help you keep your data secure while harnessing the power of AI.
How AI Puts Your Intellectual Property at Risk
Think about it: AI relies on lots of data. The more data, the better it performs, but if that data includes sensitive customer details or proprietary business information, you’re treading on thin ice. In my early experiences working with AI, I quickly realized that while the technology was powerful, it also had the potential to expose parts of my business I’d prefer to keep under wraps.
Consider this analogy: using AI is like hiring an incredibly talented contractor who’s brilliant at solving problems but has access to parts of your company that are typically off - limits. If this contractor starts copying parts of your proprietary designs or sharing sensitive data, your IP is at risk. Similarly, AI can be a double - edged sword - it’s useful but can expose critical data if you’re not careful.
Here’s why AI can put intellectual property (IP) at risk:
1. Data Volume and Sensitivity: AI models thrive on vast, often complex datasets, and many of these contain sensitive or proprietary information - anything from customer details to unique product designs.
2. Open Data Exchange: Training and testing AI models usually involve multiple platforms and systems, sometimes even third - party vendors. Every exchange can open a door to potential data leakage or unauthorized access.
3. Model Transparency Risks: Some AI models, especially machine learning models, can inadvertently reveal patterns in proprietary data. For example, when models are reverse - engineered, there’s a risk that unique insights or methods derived from proprietary data could be extracted.
4. Regulatory Compliance: With regulations like GDPR and CCPA, organizations face additional responsibilities around data usage. If proprietary data isn't handled according to these laws, businesses risk legal repercussions that can harm their reputation and bottom line.
The complexity of these risks is real. When I first began integrating AI into a project, I found myself weighing the rewards against the very real risk of exposing critical data to unforeseen vulnerabilities. For any business, striking this balance is crucial.
Strategies for Protecting Your IP While Using AI
The good news is that with the right safeguards in place, you can take advantage of AI’s capabilities without compromising on IP security.
At Engineering Insights, we apply a structured approach to AI projects using our 5D framework - Discover, Define, Design, Develop, and Deploy - to integrate IP protection from start to finish.
1. Secure Your Data from the Start
The first and perhaps most critical step in protecting IP is securing the data that feeds your AI model. A strong foundation for data security includes:
• Data Classification: Segment and classify data based on sensitivity and access requirements. This helps establish which data can be used in AI models and what needs special protections.
• Access Control: Implement strict access controls, ideally with role-based permissions. This ensures only the right people (and systems) can interact with sensitive data.
During one project, I learned the hard way about the importance of limiting data access. I hadn’t anticipated the ripple effect of certain team members having access to information they didn’t need. By setting strict access controls and monitoring who could see what, I significantly reduced this risk and increased overall security.
• Data Minimization: Avoid feeding unnecessary sensitive data into your AI model. Use only the data that is essential for the model to function effectively.
2. Embrace Data Anonymization and Encryption
While AI systems need data to perform, that data doesn’t always have to be identifiable. Data anonymization techniques help reduce the risk of sensitive information exposure by removing identifying characteristics without compromising data utility.
• Data Anonymization: Strip out personally identifiable information (PII) or other proprietary markers from your data before it enters the AI model. For example, you could replace names with numerical IDs to keep the data usable but anonymous.
• Encryption: Encrypt data both in transit and at rest to prevent unauthorized access. Using strong encryption standards, such as AES - 256, adds an extra layer of protection, so even if data is intercepted, it remains unreadable.
3. Conduct Regular Audits and Risk Assessments
AI systems are dynamic, and data is constantly changing. Regular audits help ensure that your IP protection protocols are keeping up with new challenges.
• Automated Monitoring Tools: Use automated monitoring solutions to continuously track data access and detect any unusual behavior in real - time. For instance, anomaly detection tools can flag unauthorized access or data movement, giving you a head start in responding to potential threats.
• Risk Assessments: Periodic risk assessments can reveal new vulnerabilities or outdated protections. Stay ahead by regularly reassessing where and how your IP might be vulnerable.
In one instance, I discovered an issue during a scheduled audit where data from one project was accessible to another unrelated department. This was a permissions oversight, and without regular audits, we might not have caught it. Audits, though sometimes tedious, are essential for maintaining airtight IP protection.
4. Leverage Differential Privacy Techniques
Differential privacy is a technique used to add “noise” to datasets, making it hard for outside parties to reverse - engineer data while still allowing the AI model to generate meaningful insights. This can be especially useful when using customer or sensitive data.
For example, if your AI system is analyzing customer demographics, differential privacy can ensure that individual data points are obscured while maintaining the overall trends and patterns needed for accurate predictions.
While differential privacy requires expertise to implement correctly, I’ve found that for customer - driven AI models, it’s an invaluable strategy for balancing data utility with privacy protection. It can give you peace of mind knowing that even if an outsider accessed the data, it would be nearly impossible for them to extract identifiable information.
5. Define Clear IP and Data Use Policies for AI Projects
Beyond technical safeguards, establishing clear policies around data use and IP rights in AI projects is critical. Not everyone on your team will be familiar with data protection laws or the importance of IP integrity, so set guidelines for everyone involved in your AI initiatives.
• IP Ownership Agreements: Define and document IP ownership in any collaborative AI project, especially if you’re working with external vendors or consultants. Clear agreements on data and model ownership protect you if disputes arise down the road.
• Data Use Guidelines: Set rules around acceptable data use and train employees on compliance standards like GDPR or CCPA. This also includes how to handle data after it’s been used in the AI model - whether it should be stored, anonymized, or deleted.
6. Implement a Strong IP Protection Culture Within Your Organization
A single misstep by an employee can jeopardize your entire IP strategy, so cultivating an organization - wide IP protection culture is essential. Everyone involved in AI projects should understand the importance of IP and data security.
• Employee Training: Run training sessions on best practices for data handling, particularly for employees working directly with sensitive data. Explain the risks, laws, and company policies that affect their work.
• Clear Communication: Emphasize the importance of IP in team meetings, project kick - offs, and internal communications. I’ve found that the more awareness you bring to IP protection, the more vigilant everyone becomes.
Using AI to gain a enhance efficiency doesn’t mean compromising on data protection. Embracing AI is a game - changer, but the excitement around it can lead to overlooking IP risks.
If I’ve learned anything, it’s that strong IP protection isn’t about taking the fun out of AI; it’s about ensuring that you can keep innovating without compromising what makes your business unique.
So, take it from me - balancing AI and IP protection is achievable with a bit of planning and a few essential safeguards.
In the end, protecting IP in AI isn’t a one - time project; it’s a continuous effort that evolves alongside your AI initiatives. The strategies discussed here, from secure data handling to embracing privacy - preserving techniques, will help you enjoy the full potential of AI while keeping your innovations and proprietary data under tight lock and key.
Close